What Is Analytics Engineering? (And What It’s Not)
Table of Contents
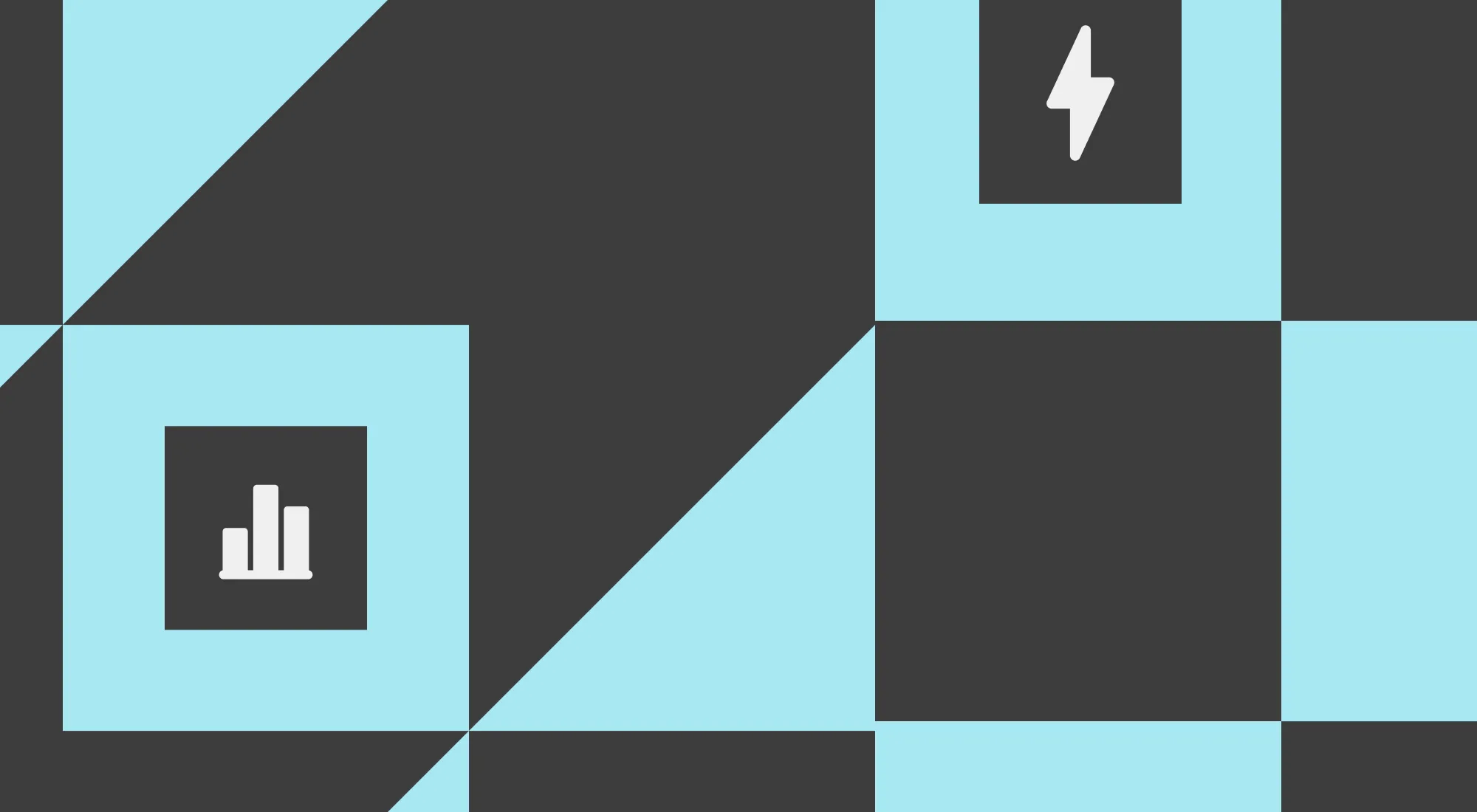
Just as changes in macro-economic markets bring about new types of jobs, changes in the field of data and analytics have brought about new tools, methodologies, and roles. One key role that emerged and was popularized by dbt Labs was “Analytics Engineer.”
Previously known by many names such as Business Analysts, Data Wranglers, or BI Developers, this role is not necessarily new, though the requirements and responsibilities for this role have been refined over the years. In this blog post, we will discuss what an analytics engineer does (and doesn’t do) and how this role can be extremely valuable to a data and analytics organization.
How did the analytics engineer role come about?
To understand why analytics engineers are so important, we must first understand how the role emerged and how we got here, so first, a history lesson.
BI's evolution: The spark that created the analytics engineer
Let’s rewind to the late 1990s and early 2000s when systems we now refer to as legacy BI were emerging in popularity. These legacy BI tools, such as Cognos, BusinessObjects, and Hyperion, were being owned and operated within central IT teams. This was standard operating procedure for the time, and it made sense because the technical knowledge and understanding of data systems were not as proliferated within the workforce as they are today.
These tools were fairly clunky and designed with IT users in mind. They generally output what we would refer to today as paginated reports for end users to either print out or export to Excel to put into a Pivot Table for further analysis.
Fast forward to 2010: a new wave of BI tools hit the market that focused on being low/no code and marketed at the line of business (LOB) users, the most notable of them, Tableau. This wave of low/no code drag-and-drop BI tools proliferated the idea of self-service analytics that allowed LOB users to create their reports and dashboards for their business processes rather than having to rely on central IT for analytics.
While self-service analytics had many upsides, many drawbacks came with it: massive training and upskilling budgets needed for users, loss of central IT governance and control over data models and usage, and duplicative work across LOBs that ultimately resulted in increased cost of ownership and time lost.
This is where the analytics engineer was born.
What is an analytics engineer?
The widespread adoption of low/no code BI tools like Power BI and Tableau created both opportunities and challenges. Organizations needed a way for Lines of Business (LOB) and central IT to work more efficiently together. Analytics engineers bridge this gap by understanding business use cases, speaking the language of IT, and having the technical skills to translate business requirements while understanding the overarching IT data strategy.
The data interpreter: Bridging the business-tech communication gap
These gaps between central IT and the users working in the lines of business are not negative but rather reflect differences in understanding, skill sets, and context. Central IT teams focus on the technical aspects of data, ingestion, transformation, and modeling in a cloud data warehouse (CDW), while LOB users focus on using data for business insights. Analytics engineers serve as the essential bridge spanning these different perspectives.
What do analytics engineers do?
Now that we’ve covered how we got here and the role of an analytics engineer, let’s dive into how this plays out in a real organization.
Gathering requirements and building data models
When a business need arises, analytics engineers meet with key stakeholders to gather requirements. With their knowledge of both the business and the CDW, they piece together a plan of action, using tools like Alteryx, KNIME, or Sigma to investigate existing data models and determine what data is needed to solve the business problem.
Collaborating with IT for data product development
Once analytics engineers craft a solution from business requirements, they can either use existing data models in the CDW or work with IT to build new models that meet business needs. They can take already existing self-service data products created by LOB users and create more sustainable solutions by integrating them with central IT systems.
This methodology ensures LOBs maintain flexibility to create self-service analytics while ensuring central IT still owns and governs the underlying data models. The analytics engineer operates more agilely than LOB users and at a lower cost than dedicated IT resources, enabling accelerated creation of data products with proper governance.
Why are analytics engineers so important?
Analytics engineers create tangible value by minimizing costly IT resource utilization while maximizing effective communication between teams.
Reducing costs and accelerating development
Generally, IT resources have higher cost rates than LOB analysts. This means that the more time that IT works on a LOB data project, the less ROI a LOB will see on that project. That logic is true for any resource, but given the high bill rate of IT resources, the impact is even greater.
Given the particular cost of IT resources compared to analytics engineers, this cost difference has a significant impact. Analytics engineers can quickly prototype and productionalize data assets at a lower cost, which not only has budget implications but also dramatically improves the timeliness of fulfilling data needs within large organizations.
Improving communication and team effectiveness
In addition to the cost advantages of having an analytics engineer working in your scrum team, analytics engineers also provide an effective component to any data team. As the bridge between teams, analytics engineers comprehend business processes and can communicate those needs to IT in technical terms they understand. This results in less development time, more accurate requirements, and better data solutions.
Analytics engineers as part of fusion teams
Analytics engineers have become such a part of the modern data organizational structure that when Gartner formalized and popularized the concept of Fusion Teams around 2018, the analytics engineer played a central role. For those unfamiliar with the concept, Fusion Teams are multidisciplinary teams that blend business and IT roles to design and deliver digital initiatives, essentially "fusing" IT and the LOB.
In these domain-centric teams, data engineers ensure relevant data is properly ingested and modeled, BI developers create needed data products, and analytics engineers quickly prototype data models and business logic to meet business requirements. This collaborative model has proven efficient, leading to lower costs for digital initiatives, faster delivery times, and increased trust in central IT's data assets.
How Sigma enables analytics engineers
An effective analytics engineer needs tools that allow them to access underlying data from the CDW, visualize data for validation and testing, and drill down to specific data points to investigate discrepancies or validate KPIs. Many tools can do some of these things, but Sigma is particularly well-suited for these unique requirements.
Sigma’s high-performing direct queries to the CDW allow analytics engineers to work with real-time, validated data. The intuitive syntax allows analytics engineers to use Sigma to easily create calculations for business logic and validation purposes.
Additionally, Sigma’s drill-through capabilities will enable users to investigate individual data points throughout a data product with ease and speed, which is necessary for the high-paced day of an analytics engineer.