How To Automate Data Validation For Accurate And Reliable Analytics
Table of Contents
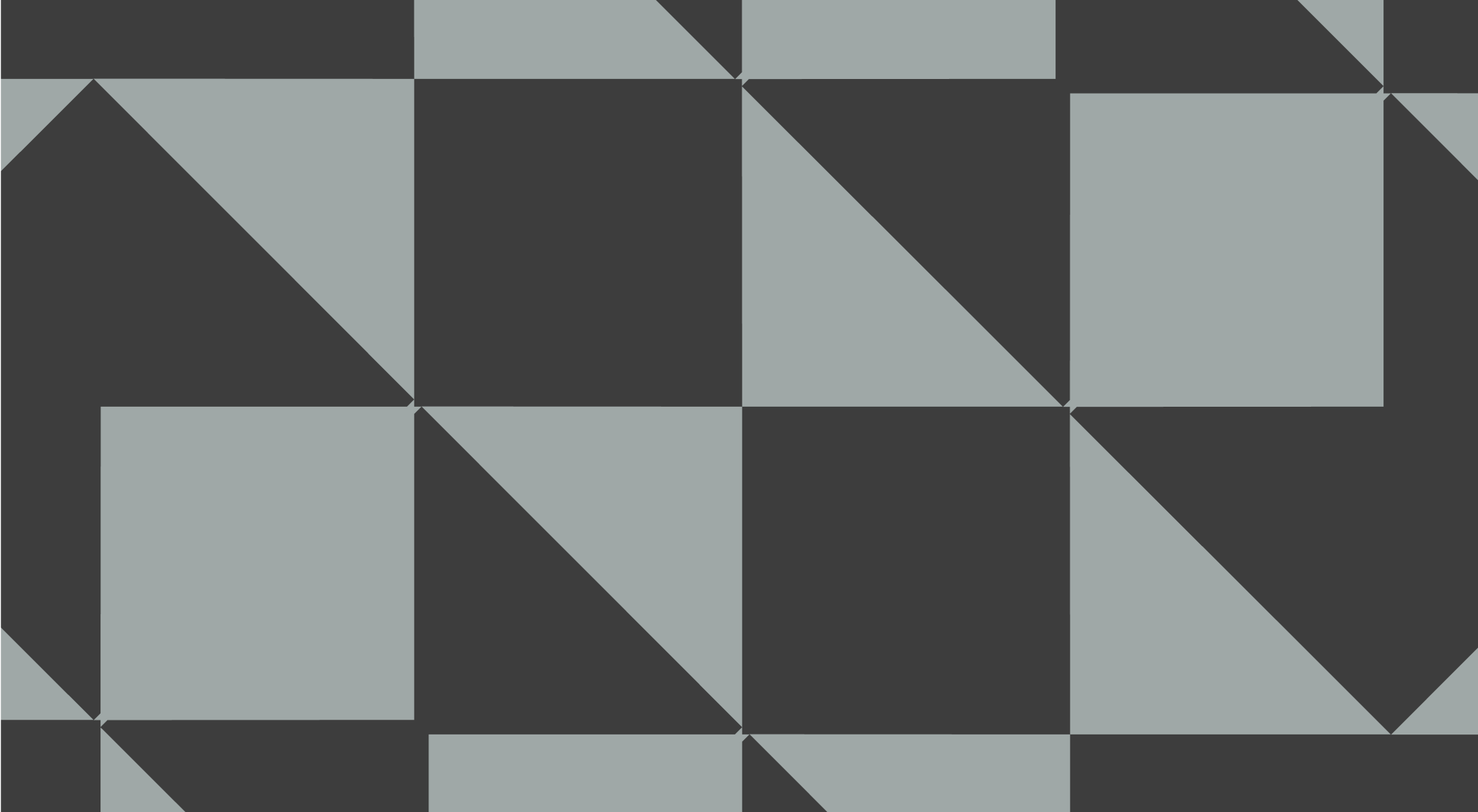
Nothing kills trust in analytics faster than a report built on bad data. Manual checks are tedious, wrought with errors, and often skipped. Let’s face it: working with messy data is exhausting. One minute you're prepping a report, and the next you're digging through a table with 36 different spellings for “California.” A misplaced decimal, a missing field, or an inconsistent date format can quietly corrupt your entire analysis.
Manual data validation is a repetitive task that quietly drains time and confidence from your analytics process. It’s easy to miss small inconsistencies, and when those issues slip through, your dashboards start telling the wrong story.
The good news is you don’t have to eyeball every cell in a spreadsheet or run the same SQL checks every morning. Automation offers a better way to catch errors early and keep your data trustworthy without the manual slog. It means folding smart checks and guardrails into the work you’re already doing, bit by bit, script by script.
Let’s walk through practical ways to automate your data validation, from writing simple scripts to setting up alerts and integrating checks directly into your ETL flows. You’ll learn what to focus on, how to get started, and how to build habits that keep your data clean and analysis-ready.
What is data validation, and why does it matter?
Data validation checks whether your data is complete, accurate, and structured consistently. At its core, it’s about making sure your data makes sense before you rely on it for decisions, dashboards, or downstream pipelines. Think of it like spell check for numbers: it won’t guarantee perfect insights, but it’ll catch the obvious mistakes before they cause bigger problems.
That might sound basic, but in reality, validation often gets skipped or rushed. When that happens, you risk working with data that doesn’t match expectations: text in number fields, nulls where there shouldn’t be any, or mismatched formats across sources. Once those issues sneak into reports or models, the damage can ripple across your team’s work.
That’s why automating validation matters. It helps catch these issues early, consistently, and without relying on someone to scan through rows or rerun filters manually. With the right checks in place, your data has a much better shot at staying clean even as sources grow and structures shift. But getting started isn’t always straightforward.
Every data source and team works a little differently, making building a reliable, reusable validation process feel like a moving target. Fortunately, you don’t have to solve everything at once. In the following sections, we’ll look at small steps that can make a big difference, starting with something you probably already use: scripts.
Why manual validation fails
Before diving into automation, it helps to understand where manual validation often goes sideways. Even if you're experienced and meticulous, these mistakes are easy to make, which makes them risky. Manual validation usually starts with good intentions: open a CSV, skim for blanks, and run a few filters. But this approach breaks down over time because the process isn’t built to last.
It’s repetitive
If you're checking the same fields for nulls daily, it becomes routine, and not the kind of routine that builds mastery. It’s mindless, manual repetition that drains time and still doesn’t guarantee clean data. When validation feels like a chore, rushing through or skipping it altogether is easy.
Humans miss things
Even sharp eyes glaze over after staring at rows and columns for too long. You can scroll through a table a hundred times and still miss that “Texas” was entered as “Txas” three times. Manual review isn’t built for scale, and the more data you handle, the more that reality hits.
Visual checks aren’t reliable
Spot-checking your data in a spreadsheet might catch glaring errors, but it rarely picks up on subtle issues. Missing IDs, bad dates, or sloppy labels don’t always stand out. Without routine checks, they’re easy to miss.
One small error can spread fast
It only takes one unchecked issue to throw off an entire report. A few bad records in a sales table? Now your forecast is off. An extra row in a lookup table? That could misclassify hundreds of customers. Bad data has a way of multiplying, and once it’s in the system, untangling it takes time you don’t have.
Manual validation doesn't scale
What works for a spreadsheet breaks down fast across databases, pipelines, and source systems. As your data grows, validation needs to keep up, and without automation, it's a matter of when, not if, something gets missed.
How to use scripts to automate data validation
Scripts are one of the simplest ways to move from reactive data cleaning to proactive error prevention. They turn manual checks into repeatable, scalable logic that runs the same way every time, with no second-guessing or missed rows.
You don’t need a massive framework to get started. A few well-placed lines of Python or SQL can catch problems early and consistently, freeing time for more valuable work. Writing scripts is the fastest way to replace manual checks with something repeatable. Here’s where to start.
How to use Python and SQL for automated data validation
Python and SQL are the go-to tools for automated validation because they’re flexible, fast, and widely supported. The goal is to create small, repeatable checks that flag problems early and reliably.
Python: Validate structure and format
Here’s a Python function that handles two of the most common issues, missing values and bad date formats:
import pandas as pd
def validate_data(df):
# Check for missing values
if df.isnull().sum().any():
raise ValueError("Missing values detected!")
# Validate date format (e.g., YYYY-MM-DD)
if not pd.to_datetime(df['date'], errors='coerce').notna().all():
raise ValueError("Invalid date format!")
print("Data passed validation.")
This check can be dropped into any data prep script. Run it once per file or per batch load; there is no need to scroll through rows to spot errors.
SQL: Surface duplicates and outliers
In SQL, validation becomes part of your query logic. For example, to catch duplicate customer records:
SELECT customer_id, COUNT(*)
FROM orders
GROUP BY customer_id
HAVING COUNT(*) > 1;
You can also look for numeric outliers or incorrect data types:
SELECT *
FROM transactions
WHERE amount < 0
OR STR_TO_DATE(order_date, '%Y-%m-%d') IS NULL;
These queries can be scheduled to run automatically or baked into your reporting logic. If anything fails the check, trigger an alert or log the result.
Write reusable functions
Rather than copying and pasting logic, wrap your validation checks in functions. This keeps your scripts clean and makes it easier to apply the same logic across datasets and projects.
Automation doesn’t have to be elaborate. Even a handful of scripts that check for common issues will go a long way toward making your data more dependable, without slowing you down.
Built-in validation functions you should know
You don’t always need to write custom code from scratch. Most databases and programming languages include built-in functions that can handle many validation tasks for you, faster and with less risk of human error. You just need to use them consistently.
SQL built-ins
- IS NULL / IS NOT NULL: Basic presence checks
- CHECK constraints: Enforce rules like non-negative values
- REGEXP: Pattern matching for things like emails or zip codes
SELECT *
FROM users
WHERE email NOT REGEXP '^[^@]+@[^@]+\.[^@]+$';
Python (Pandas)
- .isna() / .notna(): Quickly flag null values
- .duplicated(): Identify repeat rows
- .str.match(): Match known patterns
# Find rows with unexpected zip code patterns
df[~df['zip_code'].str.match(r'^\d{5}(-\d{4})?$')]
Built-in functions save time and reduce the chance of introducing errors into your logic.
Data type checks
Many platforms let you define expected data types at the schema level. Use this to your advantage. For example, if a string sneaks into a numeric column, that column might silently convert everything to text, throwing off later calculations. Checking types in your validation script can help you catch issues early.
These functions aren’t glamorous but are the foundation of effective, automated validation. Think of them as your first line of defense: easy to add, maintain, and endlessly reusable.
Scheduling automated validation scripts
Writing a validation script is a strong starting point, but running it once doesn’t get you far. To be effective, those checks should run regularly, ideally in sync with your data flow. Automating that schedule turns validation from a helpful tool into a reliable part of your daily workflow.
If you're working in a Unix-based environment, cron jobs offer a lightweight way to schedule scripts. You might run a Python script every night at midnight to scan the previous day’s data for nulls, bad formats, or duplicates. It’s a low-friction solution that works well with ad hoc scripts or smaller pipelines. Task Scheduler serves the same purpose on Windows, allowing batch files or Python scripts to run at regular intervals: daily, hourly, or whatever your system needs.
Cloud-native tools are worth considering as pipelines grow and your infrastructure becomes more complex. Options like AWS Lambda, Google Cloud Functions, and Azure Automation can run scripts in response to specific events, like a file drop or the completion of an ETL job. They’re also flexible enough to handle scheduled runs and integrate with larger workflows.
No matter how you schedule your scripts, make sure they log what they did and when. That log is more than a receipt; it's your audit trail. With it, you can catch trends over time, like a recurring spike in nulls every Monday, or a schema mismatch from a vendor file that keeps showing up. Scheduling makes validation dependable, and when your checks run automatically, they become a quiet but consistent part of your process.
How to set up alerts for data issues
Validation scripts are great at finding issues, but they’re even more helpful when you know immediately that something went wrong. A smart alerting system tells the right people, at the right time, what failed and why it matters. It moves validation from a background task into a core part of the workflow.
Start by connecting your validation checks to the tools your team already uses. For example, if a Python script spots missing values, it can send a message to Slack or fire off an email. The alert doesn’t have to be fancy. A simple message like “12 missing emails in ‘customers’ table” works well if it shows up where your team is already paying attention. But alerts only help if they’re relevant.
One or two null values in a rarely used field probably doesn’t need to interrupt anyone. That’s why it’s worth defining thresholds. Set your validation scripts to trigger alerts only when the issue reaches a level that matters. This keeps your alerts meaningful and avoids overwhelming your team.
Make your alerts specific. “Validation failed” tells no one what to do next. A better alert includes the table, column, issue, and number of records affected. That way, whoever receives the message knows where to begin or who to loop in. Whether you use Slack, email, Teams, or ticketing tools like Jira, ensure alerts land in a space your team checks daily.
Shifting to long-term, ongoing monitoring
As validation matures, alerts can shift from simple messages to ongoing monitoring. Tools like Datadog, AWS CloudWatch, and Grafana allow you to track failed checks as custom metrics. You can visualize trends over time, like a vendor file that breaks schema rules every Monday, or a data source that sends increasingly incomplete records.
When alerts are tied to incidents, the stakes are higher. Connect serious failures to systems like PagerDuty or Opsgenie to ensure they get the right attention. When possible, let alerts trigger responses automatically by pausing a load, tagging invalid records, or routing issues to QA. This creates a feedback loop that scales with your data. Even with a solid setup, habits make or break alerting. Assign ownership so alerts don’t float in limbo. Document recurring issues so your team isn’t reinventing the wheel. Review alerts regularly. If one no longer adds value or fires too often, adjust it or let it go.
The goal is to create a system your team trusts; one that highlights real problems, prompts action, and helps prevent issues from spreading.
How to automate data validation in ETL pipelines
Validation needs to be embedded at every step of the pipeline. When you automate validation within your ETL (Extract, Transform, Load) workflows, you catch problems closer to the source and prevent them from quietly surfacing in dashboards, reports, or models downstream. Start at ingestion. That’s where issues are easiest to catch and cheapest to fix. Schema checks can verify column structure before loading begins. If something looks off, you can pause the job or trigger a warning before the data spreads through your system. Record-level checks are equally important. Transactions missing required fields, invalid date formats, or mismatched values should be caught on entry. Some pipelines can afford to reject bad records; others may need to tag them for review. Either way, the goal is to isolate issues early, not clean up messes later.
Once data is loaded, transformations come into play. But cleaning data isn’t the same as validating it. Converting “NY” to “New York” might standardize a field, but that doesn’t mean every value is mapped correctly. Applying transformation rules without validation can create new inconsistencies, especially when logic changes over time. A smart approach includes checks before and after each transformation, making sure input values are as expected and output values fall within accepted ranges.
Reusable logic makes this work sustainable. Instead of duplicating transformation scripts across tables and teams, wrap standard rules into functions or dbt models. Include documentation close to the logic so others know why a mapping exists or what a specific flag means.
That’s how validation shifts from patchwork scripts to shared practice. But none of it matters without visibility. Your pipeline should log whether it ran and document what happened. A log entry that says 24,352 records processed with 17 nulls and zero duplicates tells you much more than a simple “success” message. Over time, these logs can highlight trends: a recurring spike in missing values from one vendor, or a field that starts failing quietly after a schema update.
Observability tools like CloudWatch, Datadog, or Grafana can help track those patterns. Visual dashboards make it easier to catch when something changes, and lightweight alerts can draw attention to new failures before they reach production. You can also use orchestration tools like Airflow, Prefect, or dbt to run validation steps in line with your ETL logic; automatically halting jobs when something breaks, or routing failures into queues or issue trackers.
Auditing each extract, transform, and load step helps isolate where issues occur. Storing logs somewhere accessible means analysts and QA teams can answer questions quickly without digging. Validation becomes a shared habit, not a hidden task. Automating checks throughout your pipeline improves data quality, saves time, builds trust, and gives your team confidence that what they see reflects what’s happening in the business.
3 best practices for maintaining automated data validation
Setting up automated validation is a big win. But the real value comes from keeping it sharp and ensuring it evolves with your data, systems, and team needs. These three habits can help your validation process stay relevant, effective, and easily managed over time.
1. Regularly update your validation rules
Your business changes. New fields get added, formats shift, and priorities evolve. Validation rules that made sense six months ago might not fit current requirements.
Schedule time monthly or quarterly to review your validation logic. Are you still checking the right fields? Are new data sources introducing patterns you haven’t accounted for? Treat these reviews like maintenance, not emergencies. A little attention upfront prevents broken dashboards and frustrated analysts later.
2. Test and refine your automation process
Validation scripts and alerts should be treated like any other part of your codebase; they need testing and updates. Introduce sample failure cases into staging environments to ensure your logic works as expected. And when something slips through? Go back and ask: why didn’t the check catch it? That’s a chance to fine-tune your logic and make it more resilient. Every missed issue is an opportunity to improve coverage, not a reason to throw the system out.
3. Combine multiple validation methods
No single approach catches everything. Scripts are great for structured logic. Alerts help surface urgent issues. ETL-level checks catch structural and transformation-related problems. Dashboards help you monitor ongoing patterns.
The strongest validation setups blend these layers. For example:
- Use SQL or Python for field-level rules
- Configure alerts for critical failures
- Add dashboards to spot trends
- Run automated tests inside your ETL or orchestration tool
Building gradually across layers helps your data stay clean, even as complexity grows.
Data validation automation learnings
Clean data doesn’t happen by accident. It’s the result of small, intentional steps that build up over time. Automating your validation process doesn’t need to start with a full-scale overhaul. It can begin with a simple script, a few well-placed alerts, or a quick schema check at the point of ingestion. The value shows up in the details: fewer errors, faster debugging, and more confidence in the numbers your team works with every day.
By embedding validation into your scripts, pipelines, and workflows, you're doing more than just cleaning data. You're building a system that checks itself, flags issues early, and keeps things running without relying on constant oversight. And as your data grows in volume and complexity, that reliability matters more than ever. So start small. Choose one part of your workflow that gives you trouble, and build a check for it. Let that win fuel the next one.
Because the goal is trustworthy data you can work with, build on, and actually believe in.