How To Make AI Features Work Better In Data Analysis Platforms
Table of Contents
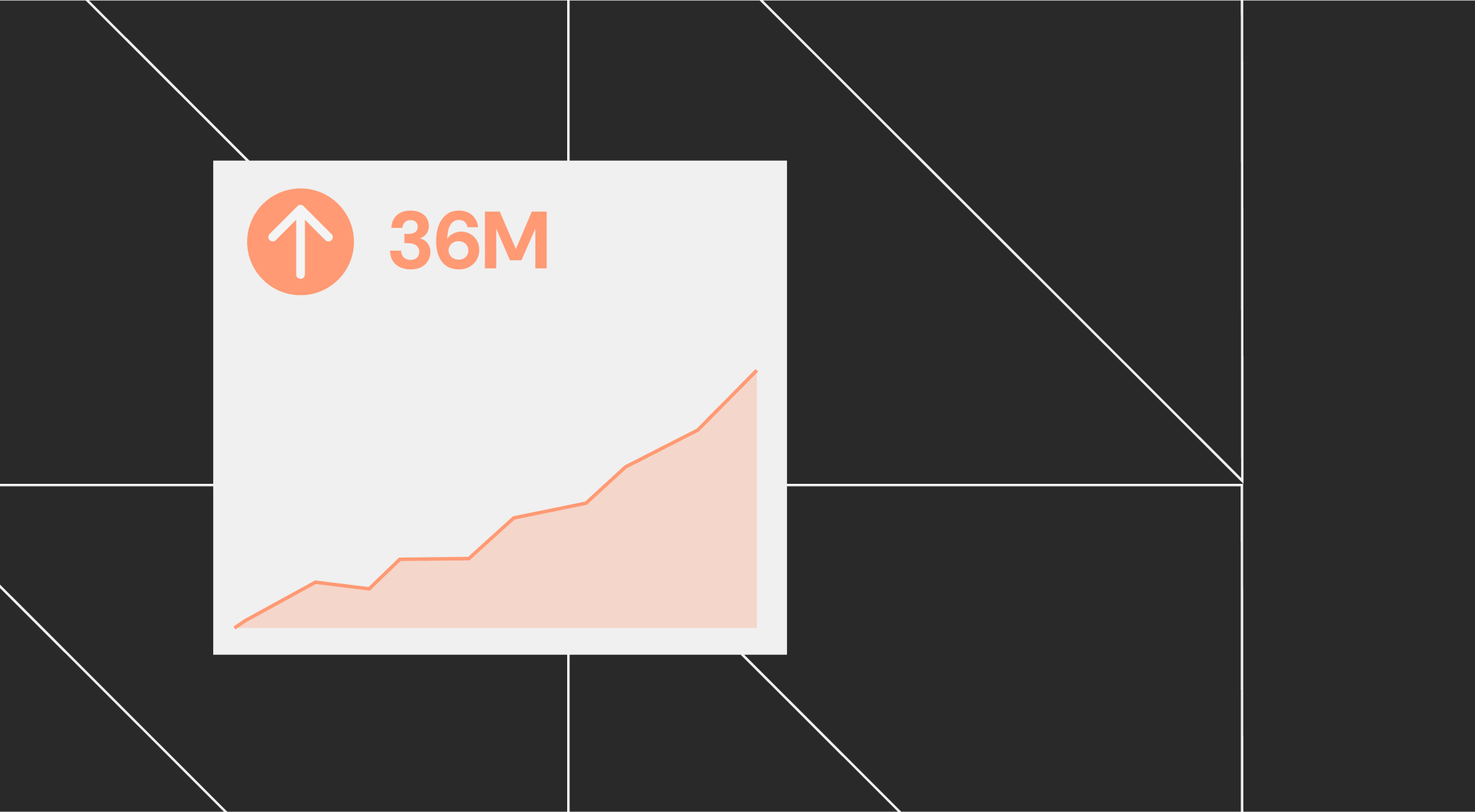
Artificial intelligence has made its way into nearly every corner of data analysis, promising faster insights and automation that improves decision-making. But for all the excitement, AI analytics features aren’t magic. They are only as good as the data they rely on and the teams using them.
If AI-generated insights aren’t delivering the clarity or accuracy you expected, the problem likely isn’t the technology itself. The real issue often lies in how the data is structured, how teams interact with AI tools, and whether the right support systems are in place to keep models performing at their best.
This blog post explains what it takes to get AI-powered analytics to work in real business settings. From data preparation to user adoption and ongoing improvements, we’ll focus on practical steps that make AI more than just a flashy feature and turn it into a tool that delivers value.
Better data preparation for AI and ML success
AI analytics tools are only as effective as the data they process. If the foundation is weak, the results will be too. Preparing data for machine learning and AI-powered analysis requires more than just gathering large datasets. It’s about ensuring the data is structured, accurate, and relevant so models can generate insights that businesses can use.
What makes data AI-ready?
AI analytics features only work when the data behind them is properly structured. Even the most advanced models struggle to deliver useful insights without the right preparation. Here’s what organizations need to focus on:
- Accuracy and consistency: A retail company analyzing customer demand needs consistent sales data. If one dataset logs prices with tax and another without, AI models might incorrectly forecast revenue or inventory needs.
- Volume considerations: In financial fraud detection, too much data can slow model performance, but too little can cause it to miss fraudulent activity. The right dataset size balances precision and speed.
- Structure and labeling: Healthcare organizations using AI for diagnosis must label medical records correctly. An unstructured dataset could lead to models misclassifying conditions, reducing reliability.
- Update frequency: AI-driven supply chain tools need regular data refreshes to account for shifting demand, weather disruptions, and supplier delays. Outdated data leads to costly miscalculations.
- Governance and compliance: AI models used in hiring must follow strict regulatory guidelines to prevent bias. Clear governance frameworks help ensure AI decisions are ethical and defensible.
Skipping these steps can result in models that misinterpret data, produce misleading recommendations, or fail to adapt as business needs change.
How to make data nerds love AI/ML analytics features
AI analytics tools don’t just need high-quality data. They need people who trust and know how to use them. Without the right training and support, even the most advanced AI models become frustrating roadblocks instead of helpful tools. The key to success is making AI a natural part of daily workflows by ensuring teams have the knowledge, resources, and systems to use it effectively.
Training programs that make AI less of a mystery
Throwing users into AI-powered dashboards without guidance leads to frustration. A well-structured training program ensures teams understand how AI processes data, what affects its outputs, and when human judgment is needed.
For example, a marketing analytics team using AI to predict customer churn might dismiss the model’s insights if they don’t understand what factors drive its decisions. Suppose they aren’t trained on how variables like purchase history, engagement levels, and seasonality impact predictions.
They may override useful AI-driven insights with gut instinct instead. Training should walk through how the model works, what data it relies on, and how confident its outputs are so teams can trust and apply its recommendations.
Support systems that keep AI in use
Even well-trained users encounter challenges. AI models shift, new features roll out, and business needs evolve. Without ongoing support, AI tools often become underused or misapplied.
Companies prioritizing AI adoption often provide direct access to AI analysts or data support teams. For instance, a global logistics firm using AI for route optimization benefits from a dedicated expert who can adjust models when weather patterns, fuel costs, or demand change. Without this support, frontline employees may fall back on manual planning, reducing the tool’s value.
Create documentation people will actually use
No one has time to sift through a hundred pages of technical documentation. AI tools should have clear, searchable guides that explain concepts in simple language with real-world scenarios.
A fraud detection system in a financial institution, for example, should include practical case studies that walk users through why a flagged transaction was considered risky. Instead of drowning teams in machine-learning terminology, effective documentation should provide straightforward explanations that help users make informed decisions.
Offer feedback loops that keep AI models relevant
AI models aren’t static. They need human input to refine predictions and adjust to shifting trends. Encouraging teams to report inaccuracies or inconsistencies allows AI to improve over time.
A retail chain using AI for demand forecasting, for example, should have a way for store managers to flag when predictions are significantly off. That feedback should trigger a review to improve how the model accounts for regional demand, unexpected weather changes, or local events. Users who see their input reflected in AI recommendations are more likely to trust and rely on the technology.
Continuous improvement in AI/ML deployments
AI models aren’t set-it-and-forget-it solutions. They become less effective over time without regular updates, performance monitoring, and fine-tuning. Continuous improvement ensures AI remains relevant, accurate, and aligned with business goals.
Performance monitoring keeps AI from going stale
AI models perform well at first, but their accuracy can decline as business conditions, customer behaviors, and external factors change. Regular performance checks help catch issues before they impact decision-making.
For example, a bank using AI for loan approvals needs to assess whether its model is still making fair and accurate predictions. If approval rates shift unexpectedly or the model starts favoring certain demographics, adjustments may be required to prevent unintended bias.
User feedback turns AI into a learning system
AI should adapt based on how people interact with it. Collecting user feedback, especially those making data-based decisions, helps refine models to better meet business needs.
A sales team using AI-generated forecasts might notice that predicted deal closures don’t match actual outcomes. Their input can drive improvements if the model relies too heavily on historical data without accounting for current market shifts. Creating an easy way for users to submit feedback ensures AI evolves alongside business realities.
Retraining AI models to reflect new patterns
Data shifts over time, and AI needs fresh information to remain accurate. Regular model retraining prevents outdated predictions and improves long-term performance.
A retailer using AI for inventory management might see purchasing patterns change due to seasonality, economic shifts, or supply chain disruptions. If the AI isn’t retrained on new data, it could continue suggesting stock levels based on outdated demand patterns. Automating retraining schedules based on data quality assessments ensures AI remains reliable.
Governance frameworks keep AI in check
AI models need oversight to prevent unintended consequences. A structured governance framework ensures AI operates within ethical and regulatory boundaries while maintaining business value.
For example, an insurance company using AI for claims processing must regularly audit its models to ensure compliance with industry regulations. This includes checking for biases, assessing decision accuracy, and adjusting to stay within legal and ethical guidelines. Establishing internal policies for AI governance protects both the business and its customers.
Prepare to succeed with AI and machine learning
AI-powered analytics can transform how businesses operate, but they only work when the data and the people using them are set up for success. Poor data quality, lack of user adoption, and outdated models are the biggest roadblocks to getting real value from AI.
Organizations can ensure AI-driven insights remain relevant, accurate, and actionable by focusing on better data preparation, strong user adoption strategies, and continuous model improvement. High-quality data provides a strong foundation, while training, support, and feedback loops make AI tools easier to trust and use. Meanwhile, ongoing monitoring and governance frameworks help AI models stay aligned with business needs over time.
The companies that see the most success with AI aren’t just plugging in models and hoping for the best. They actively manage AI performance, ensure teams know how to interpret and apply insights and refine models as business conditions evolve. AI isn’t a magic fix, but it can become an essential tool for better decision-making with the right strategy.