How Unified Business Analytics Tools Offer Clearer Data Decisions
Table of Contents
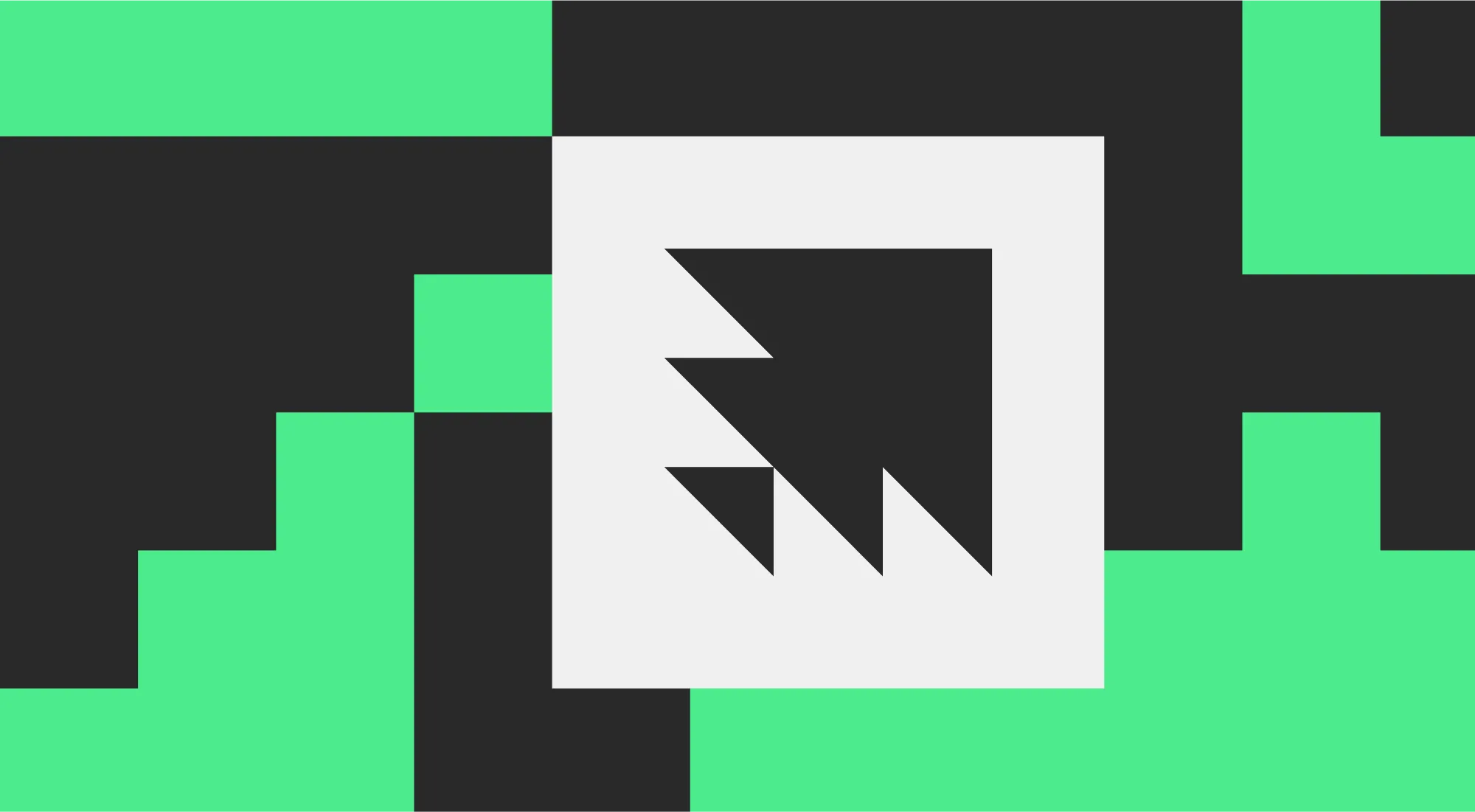
In many organizations, between CRMs, marketing platforms, ERP systems, and external tools, data is scattered across multiple systems that rarely communicate effectively. Instead of empowering teams, this fragmented data often causes more problems than it solves: slow decision-making, inconsistent reporting, and missed opportunities.
Data integration solves this by unifying data into a single, accessible system, creating a foundation for real-time insights and informed decisions. Unlike fragmented data, integrated systems help you see the full picture, streamline operations, and enable teams to respond quickly to new opportunities.
For data leaders, unifying data goes beyond technical upgrades as a competitive necessity. Organizations with cohesive, reliable data are faster, more agile, and better positioned to adapt to changing markets. Here, we’ll focus on what it takes to unify your data: the key challenges, essential components, and the features that give businesses a strategic edge.
The real cost of data silos
Data silos and disconnected tools are an operational nuisance that represents a significant barrier to organizational efficiency and growth. From financial losses to missed opportunities, the impact of fragmented data is far-reaching and compounding.
Companies spend heavily on redundant software licenses, fragmented data storage, and manual reconciliation processes, all of which inflate operational expenses. Research suggests these inefficiencies can drain up to 20% of annual revenue that could be better spent on strategic initiatives.
The time lost to manual processes compounds these financial losses. Teams frequently spend hours merging data from different systems, delaying insights that drive decision-making. For instance, finance teams might waste days consolidating reports from separate accounting, sales, and inventory systems, leaving little bandwidth for proactive planning. This reactive approach to data limits organizational agility and prevents leaders from focusing on high-impact activities.
Silos also create incomplete insights that can derail growth. Without a unified view, teams make decisions based on partial or outdated information. Marketing might analyze campaign performance, but sales may miss critical customer feedback, leading to missed opportunities for upselling or market adaptation. Fragmented data hinders collaboration and prevents organizations from leveraging their full potential.
Beyond inefficiencies, silos introduce risks to security and compliance. Managing access controls and ensuring regulatory adherence across disconnected systems is challenging. Inconsistent governance leaves organizations exposed to data breaches and penalties.
The impact on customer experiences is equally concerning; incomplete customer profiles mean missed personalization, retention, and long-term loyalty opportunities. The combined financial, operational, and strategic costs of silos underscore the urgent need for unified data integration.
Common data integration challenges
While the benefits of unifying data are clear, organizations often encounter significant challenges when integrating their systems. These obstacles stem from technical, operational, and organizational factors, making integration complex. Here are some of the most common roadblocks:
Breaking free from legacy systems
Legacy systems are one of the biggest hurdles to data integration. Older platforms were not designed to connect with modern tools, leading to compatibility issues that require custom solutions or costly replacements. Similarly, inconsistent data quality creates challenges during integration. Duplicate records, incomplete entries, and varying formats slow the process and reduce the reliability of the output.
Real-time versus batch processing: which is better for your organization?
Another significant challenge is managing real-time versus batch processing requirements. Organizations need to balance the demand for instantaneous updates with the scalability and cost-effectiveness of batch processing. Security and access control further complicate the picture, as integrating systems often exposes vulnerabilities and requires robust encryption and governance protocols.
Cross-collaboration challenges can cause barriers
Beyond technical barriers, organizational challenges also come into play. Integration efforts often require collaboration across departments, which can be difficult when teams have differing priorities, tools, or workflows. These barriers underscore why many organizations delay or struggle with unifying their data.
These challenges underscore the importance of selecting tools that address technical requirements and align with organizational workflows and goals.
Key capabilities of modern data integration
Creating a seamless data integration strategy requires key technologies and features that address both the technical challenges and business needs of modern organizations. These key capabilities form the backbone of successful integration strategies:
Foundational technologies
Data warehousing solutions: Data warehousing and lake solutions are at the heart of any unified data ecosystem. These platforms, like Snowflake or Amazon Redshift, act as centralized repositories for structured and unstructured data, allowing businesses to consolidate information from diverse sources while ensuring scalability and high performance.
ETL capabilities: Equally important are Extract, Transform, Load (ETL) capabilities, which automate data extraction from disparate systems, transform it into a standardized format, and load it into centralized storage. These tools reduce manual effort and ensure that teams work with clean, reliable data ready for analytics and reporting.
API management: Complementing this is API management and connectivity, which bridges the gap between legacy systems, third-party applications, and modern cloud platforms, enabling seamless data flow and reducing compatibility issues.
Real-time processing: For businesses requiring real-time insights, real-time processing engines are indispensable. These tools process and analyze data as it is created, empowering organizations to act on information instantly. This is a critical capability for industries like e-commerce and finance, where agility can drive significant competitive advantages.
However, ensuring this data is accurate and trustworthy requires data quality and governance tools. Features such as validation, profiling, and audit trails help organizations maintain compliance and build confidence in their analytics.
Platforms like Sigma enhance this process by providing direct connectivity to cloud data warehouses, enabling teams to analyze and collaborate on unified datasets without complex data extractions.
By combining these components, organizations can build integration systems that unify data and enhance its accessibility, usability, and security.
Value driving features
To stay competitive in a fast-moving business environment, organizations must adopt data integration tools with features that do more than unify data – they must enable agility, scalability, and strategic decision-making. Here are the key features Leaders should prioritize when evaluating integration solutions:
High-value data quality management
The foundation of any effective data integration system is reliable data. Tools that offer robust profiling and validation ensure that datasets are clean, consistent, and ready for analysis.
Cleansing and standardization processes prevent errors from propagating across systems, while duplicate detection eliminates redundancies that can skew results. Additionally, error handling and logging features provide visibility into integration issues, helping teams address them proactively.
Industry-standard security and compliance
Security and compliance features are non-negotiable. Data encryption protects sensitive information during transfer and storage, while access controls ensure only authorized users can interact with critical data.
Audit trails and compliance monitoring help businesses meet regulatory requirements such as GDPR and HIPAA, and privacy protection measures like anonymization safeguard personal data.
Automation capabilities for agility
Automation is key to maintaining flexibility and efficiency in data integration. Workflow automation reduces manual tasks, enabling teams to focus on strategic initiatives. Scheduling tools ensure data updates happen consistently, while real-time monitoring and alerts provide insights into performance and potential issues.
These features allow organizations to adapt quickly to new demands without sacrificing quality or speed.
Scalability features to support growth
As businesses expand, their data integration tools must scale seamlessly. Performance optimization features maintain speed even as data volumes grow, while resource management ensures efficient use of computing power.
Load balancing prevents bottlenecks during peak usage, and caching mechanisms accelerate queries to deliver faster results. These capabilities allow organizations to handle growing demands without overhauling their systems.
These features collectively address critical pain points, enabling businesses to integrate, secure, and scale their data systems effectively.
What’s next for data integration?
As businesses continue to adapt to new challenges, the field of data integration is evolving to meet emerging needs. Understanding these trends can help organizations future-proof their strategies and stay ahead of the competition. Unifying data involves addressing critical challenges, implementing essential technologies, and leveraging advanced features to ensure agility, scalability, and security. By embracing integration, organizations can break down silos, streamline operations, and unlock the full potential of their data to drive strategic decision-making.
One of the most transformative developments is integrating artificial intelligence (AI) and machine learning (ML) into data workflows. These technologies automate data mapping and anomaly detection processes while enabling predictive analytics. Machine learning algorithms can now optimize ETL workflows dynamically, saving time and enhancing accuracy.
Real-time processing engines are also advancing to meet the growing demand for instantaneous insights. Retail, finance, and healthcare industries rely heavily on up-to-the-second information to remain agile in fast-paced environments. With real-time tools becoming more powerful, businesses can now process and analyze massive datasets almost instantaneously, fundamentally redefining expectations for speed and responsiveness.
In parallel, low-code and no-code platforms make data integration more accessible to non-technical users. These tools empower business teams to design workflows without relying on IT, reducing bottlenecks and accelerating project timelines. This helps organizations adapt to new opportunities faster while freeing up technical resources for more complex tasks.
By combining foundational integration practices with these emerging trends, businesses can ensure their data strategies are future-proofed, resilient, and aligned with market demands. The key to long-term success lies in leveraging integrated data to respond to today’s challenges but to anticipate tomorrow’s opportunities.