What If Your Data Could Think for Itself? A Beginner’s Guide to Edge Analytics
Table of Contents
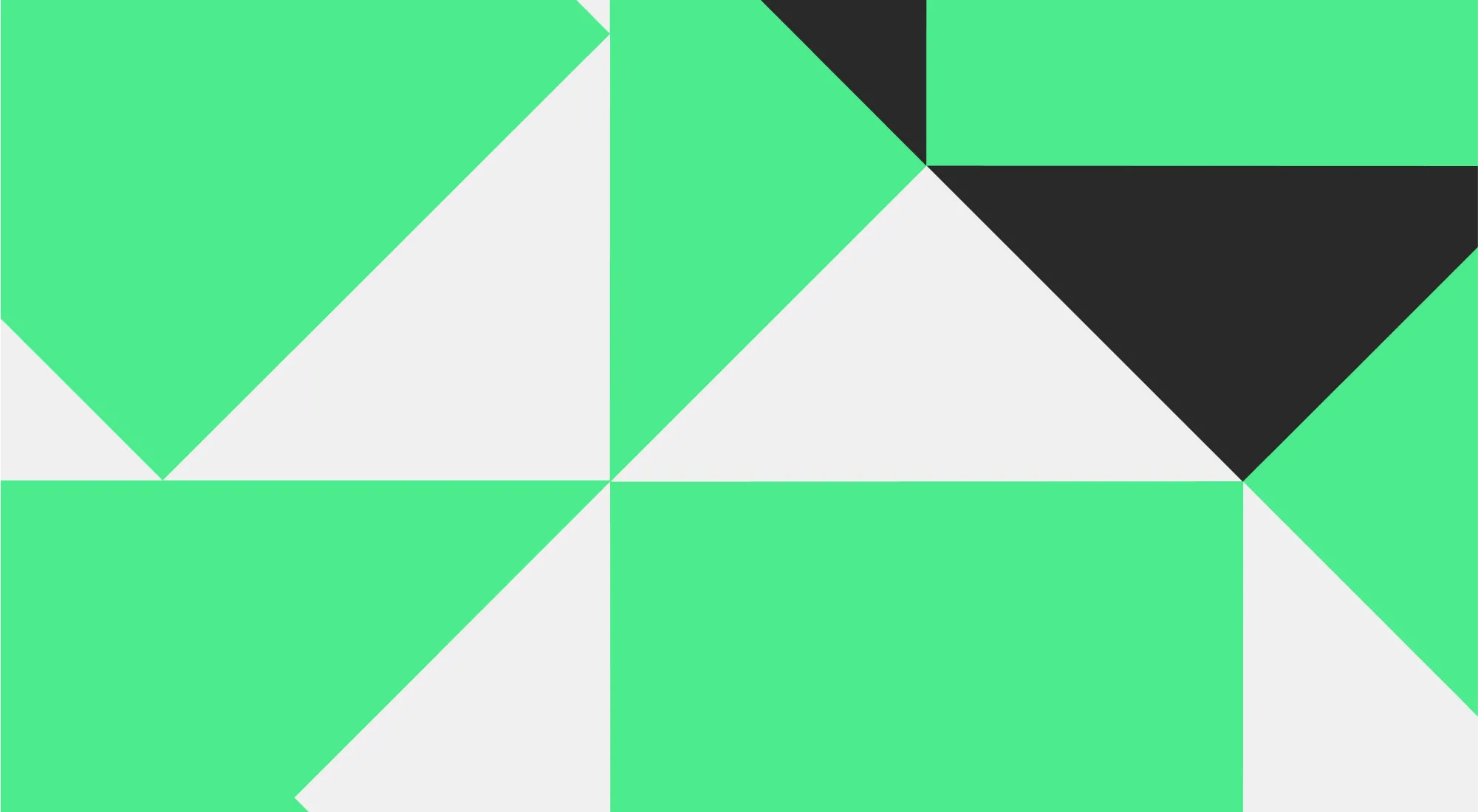
When a traffic light adjusts its timing as cars approach or a factory sensor detects a vibration and triggers a repair request before a breakdown occurs, you are seeing analytics close to the action.
Most analytics still happen far away from where data is created. Data gets sent to cloud servers or central systems for processing, and results come back seconds later. That delay might not matter when reviewing a monthly sales report. But those extra seconds could make all the difference in healthcare, transportation, or manufacturing. As connected devices become more common and expectations around speed increase, a different model has started to take shape: edge analytics. This approach skips the back-and-forth and lets data be analyzed right where it’s created: on the device or nearby.
Let’s discuss how edge analytics works, where it is being used today (from hospitals to highways), and why it's becoming impossible to ignore.
What is edge analytics?
As mentioned above, most analytics happens in one of two places:
- On-premise servers (old school, bulky, limited)
- The cloud (scalable and remote, with lag and bandwidth costs)
Edge analytics adds a third option: analyzing data where it's collected. Think of a factory sensor detecting defects and triggering action without sending video to a distant server. The “edge” refers to devices or nodes on the outer boundary of a network: the place where digital systems interact with the physical world.
Edge analytics can even function without internet access. That can make a difference in settings where connectivity is limited, or when reliability and speed are more important than pushing every data point to the cloud.
Here’s how edge analytics compares to other analytical models:
One of the biggest ideas behind edge analytics is decentralization. Instead of one central hub analyzing everything, decisions are made closer to the source. That’s valuable when systems need to respond quickly, or when privacy concerns limit how data can be shared.
Where is edge data collected and processed?
Edge analytics is often associated with IoT gadgets but goes far beyond smart thermostats and fitness trackers. Additionally, retail stores are already using edge analytics to manage inventory in real time. For example, smart shelves equipped with RFID sensors can detect when items are running low and alert staff before stockouts happen. Large chains like Kroger have piloted this approach to improve shelf availability and reduce lost sales.
The pattern is the same in all these scenarios: data is created and processed close to the action. Think about how that works: when a security camera detects motion, its built-in processing checks for recognizable threats. If something looks suspicious, it sounds an alarm. At that time, it also sends a relevant clip to the cloud. That “filter first” model means systems don’t waste time or bandwidth transferring hours of footage; only sharing what matters.
The edge includes a range of locations and hardware: embedded sensors in industrial machines, mobile and wearable devices, vehicles equipped with onboard computing, and edge gateways deployed near the source of activity. Edge servers or micro data centers support more demanding workloads in some setups. These devices may coordinate through fog computing or mesh networks, where processing tasks are distributed and local collaboration replaces reliance on centralized control.
The use of embedded AI
Additionally, many edge systems also use embedded AI to run lightweight machine learning models. Instead of waiting for a remote model to analyze incoming data, the device makes decisions independently, adjusting, alerting, or reacting in real time. This approach is helpful in disconnected or high-latency environments, like rural clinics or underground mining operations, where quick response matters more than a full data sync.
The value here is speed and control. By collecting, analyzing, and acting on data at the edge, systems become more efficient, responsive, and autonomous. Because less data has to travel, organizations save on bandwidth and improve privacy, too.
Why organizations are making the switch
Moving data processing closer to where it’s created makes things faster and changes what’s possible. When systems can react immediately, stay online even without a connection, and limit how much raw data leaves the device, a lot of things get simpler.
Here’s what that looks like in practice:
Speed means survival
In manufacturing, every second counts. Waiting for a cloud-based system to catch a quality issue can mean piles of defective products before anyone notices. With edge analytics, sensors spot defects in real time, helping teams adjust immediately and reduce waste. In finance, edge-powered fraud detection systems flag suspicious transactions before they even complete, blocking potential damage before it starts.
Lower bandwidth, lower costs
Factory floors and industrial sites generate massive amounts of data, but most of it doesn’t need to leave the building. Edge systems filter out routine readings and only transmit anomalies. That shift cuts down on data transfer and lowers cloud storage bills without sacrificing insight.
Privacy stays local
In healthcare, edge analytics allows hospitals and clinics to keep sensitive data on-prem. That makes it easier to meet privacy regulations while still getting the insights needed to care for patients. Instead of routing medical scans or vitals through third-party systems, analysis can happen directly on secure local infrastructure.
Always on, even offline
Edge analytics doesn’t break when the internet does. On remote oil rigs or rural wind farms, edge systems monitor, detect issues, and keep operations running even when satellite connections drop. That reliability means less downtime and fewer interruptions in places where repairs aren’t easy.
How edge analytics supports emerging technologies
As new technologies develop, they demand more from data systems like faster responses, smarter automation, and stronger reliability. Edge analytics fills those gaps by helping these systems process information on the spot instead of waiting for a cloud or centralized setup to weigh in.
Take connected vehicles, for example. From navigation to collision avoidance, edge analytics lets systems inside the car process data on the spot. There’s no time to send sensor readings to the cloud and await a response. Braking decisions need to happen in milliseconds.
That same local processing supports fleet management too, allowing logistics teams to monitor vehicle conditions and routes in motion without relying on a stable connection. In industrial automation, edge analytics strengthens IoT networks by helping machines and sensors communicate and adapt in near real time. Factories can coordinate production lines, detect early signs of equipment failure, and adjust outputs based on current demand. The result is a setup that reacts, optimizes, and stays resilient even when networks falter.
5G is also accelerating adoption. With lower latency and higher capacity, 5G networks make pushing more processing to the edge easier. That makes a difference in everything from smart city infrastructure, such as adaptive traffic lights or real-time public safety alerts, to augmented and virtual reality applications that rely on fluid, low-lag responsiveness.
In education, schools are exploring edge analytics for building safety and smarter classrooms. Systems that adjust lighting, HVAC, or digital displays based on real-time occupancy can reduce energy use and enhance learning environments.
Artificial intelligence is also moving to the edge. Devices now have the processing power to run trained models on their own. This allows tools like diagnostic scanners, surveillance systems, or industrial robots to respond immediately based on past patterns, not just pre-programmed instructions.
The common thread in all these is fast feedback loops and local intelligence. Edge analytics supports the responsiveness and autonomy these systems need to work at scale.
The future of edge analytics
Edge analytics is moving beyond early adoption. What’s next are systems that respond locally and learn and adapt independently. Traffic lights that fine-tune timing based on daily patterns. Wind turbines that schedule their own maintenance before technicians even run diagnostics. These aren’t science fiction. They’re already being tested and quietly deployed.
The shift isn’t just about making things faster. It’s about making entirely new types of decisions possible. Local processing unlocks autonomy in places where sending data back and forth simply isn’t practical. It opens the door for smarter cities, adaptive infrastructure, and systems that improve through experience, not just input. As data volumes surge, sending everything to distant servers is becoming outdated. The real question isn’t whether edge analytics will play a role; it’s where you’ll use it first.