How Statistical Modeling Transforms Raw Data Into Business Gold
Table of Contents
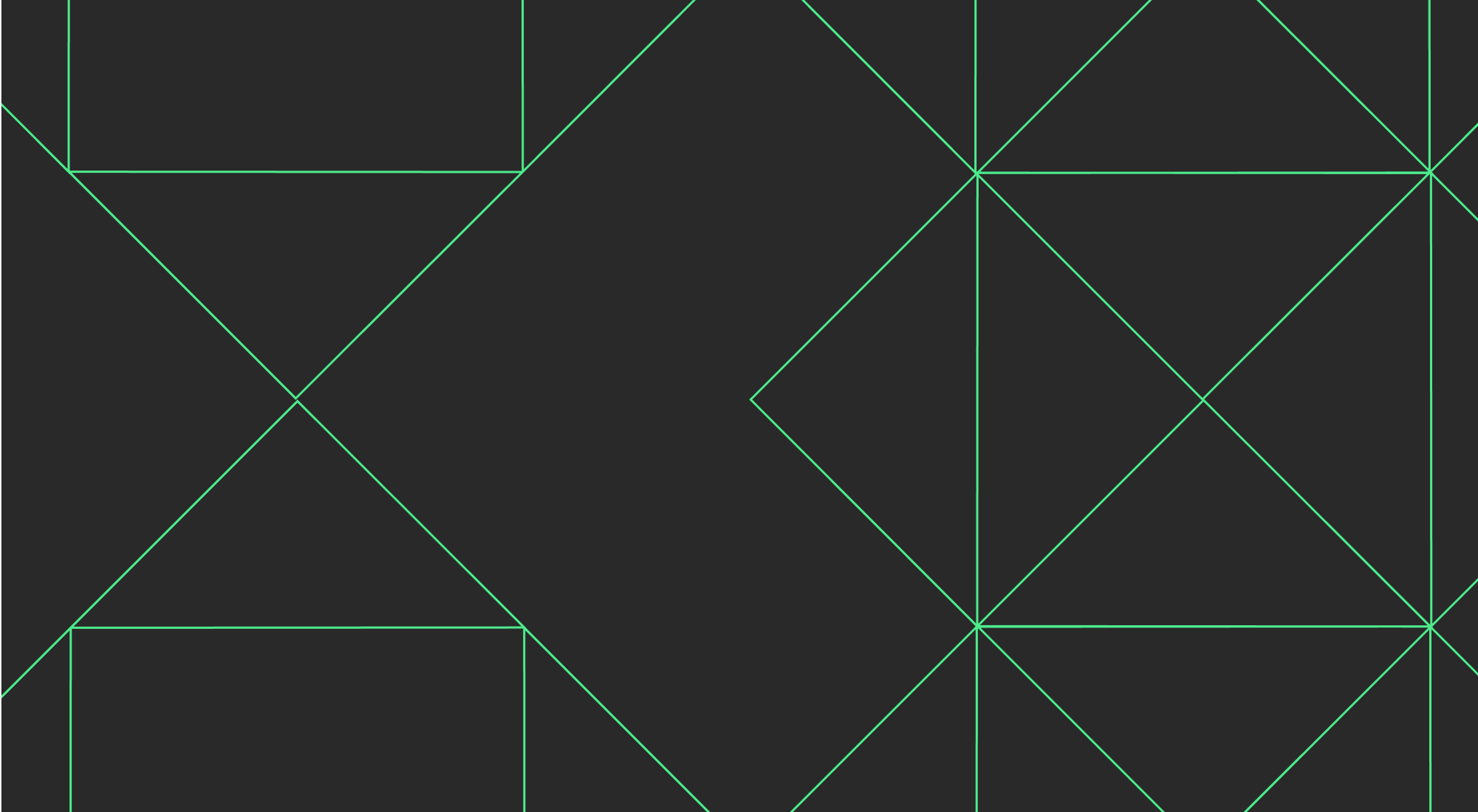
Imagine sitting on a mountain of raw data, but no real way to know what it’s trying to tell you. You can feel there’s a story buried in the numbers, but it’s not speaking your language. Statistical modeling helps translate messy datasets into patterns, probabilities, and predictions you can use.
For data teams, statistical modeling is often the point where curiosity turns into clarity. Instead of relying on guesses or instincts, you’re using logic and probability to ask better questions and get answers you can trust. Statistical modeling isn’t just about forecasting what might happen. It helps you understand why things happen and what to do next. That’s what turns a spreadsheet into strategy.
Let’s walk through modeling, its techniques, and how companies across industries apply it to real problems. We’ll also cover how to make sense of the results and what to watch out for when things get complicated.
How to enhance decision-making with predictive insights
Decisions are everywhere: what to build, who to target, how much to spend. But the best decisions don’t just come from experience or instinct. They come from spotting patterns early and planning accordingly. Think of it like a weather report: meteorologists take historical weather patterns and current conditions to predict tomorrow’s forecast, and businesses do the same thing.
By combining historical data with statistical modeling techniques, companies can predict what’s likely to happen next, allowing them to plan proactively rather than reactively.
Regression analysis is a powerful tool for this, showing how different factors influence each other. For example, just like how rainy days really do increase umbrella sales, regression helps businesses understand the relationships between variables, such as how marketing spend influences sales or how seasonal factors affect product demand.
Similarly, time series models identify patterns over time. Think about how certain industries see demand spikes during specific months or seasons. You wouldn’t expect to sell snowblowers in July, right? Predicting these seasonal trends can help businesses prepare ahead of time.
Predictive analytics doesn’t stop with numbers; it also helps companies classify and segment data. Classification models sort customers into meaningful groups based on their behavior or demographics. For example, you might identify the “early holiday shoppers” versus the “Christmas Eve panic buyers.”
This lets businesses target their marketing efforts more precisely, increasing conversion rates and improving customer engagement.
The real shift is this: You stop reacting and start preparing. You trade fire drills for forecasts, which changes everything.
Whare are some real-world applications of statistical modeling?
The beauty of statistical modeling is that businesses across industries use it to solve real, tangible problems every day. Predictive models remove the guesswork from decision-making and allow companies to act proactively.
In retail, predictive modeling helps businesses forecast demand more accurately. Think about how retailers prepare for the holiday rush. Instead of relying on past sales data alone, they use predictive models to predict trends, stock the right amount of inventory, and optimize pricing strategies. This reduces waste, prevents stockouts, and improves customer satisfaction.
In SaaS, predicting customer churn is critical. By analyzing user activity patterns, predictive models can highlight which customers are at risk of leaving, allowing businesses to take preventive actions.
Finance is another field where predictive modeling shines. Banks and insurance companies use it to detect fraud, assess risk, and predict loan defaults. By analyzing transaction patterns or customer behavior, they can flag suspicious activity or adjust credit offerings based on a customer’s financial trajectory.
Predictive modeling is already helping organizations stay ahead of the curve by anticipating trends, reducing risks, and improving customer experiences, all of which directly impact their success.
Bridging the gap between data and business outcomes
As shown above, data is only valuable when it leads to action. Even the most accurate predictions can become unclear without the right insights to make sense of the data. This is where statistical modeling becomes essential. It helps businesses bridge the gap between raw data and real-world outcomes by turning complex datasets into actionable insights.
Statistical models can take huge amounts of data and simplify it into clear trends. For example, if product sales suddenly drop, statistical modeling can help pinpoint the cause, such as seasonal trends, shifts in consumer behavior, or adjustments in a competitor’s pricing strategy.
The real power of statistical modeling lies in its ability to tell a story. It’s not just about generating reports or dashboards; it’s about making data speak the language of business. Once you’ve gathered insights from your data, visualizing them easily for teams and leadership to understand is key. Well-designed dashboards and reports turn complex outputs into digestible narratives, helping businesses move from insights to action with clarity.
Statistical modeling removes the uncertainty between data and decision-making. The goal is to transform the overwhelming amount of raw data into clear, digestible insights that drive results and actions.
Overcoming common challenges in statistical modeling
While statistical modeling offers tremendous value, there are common roadblocks that can undermine its effectiveness. One of the most frequent pitfalls is the “Everything but the Kitchen Sink” approach. Incorporating too many variables can overwhelm the model and lead to irrelevant results.
Often, key factors like the day of the week or a specific customer behavior will have more impact than dozens of other metrics combined. The challenge is knowing which data is significant and not getting lost in an overcomplicated model.
Another common issue is the black box problem, where the model’s inner workings aren’t transparent to the people using it. When stakeholders can’t understand how a model arrives at its conclusions, trust in it diminishes. This was the case with a bank’s loan approval system, which was ultimately scrapped because even its creators couldn’t explain certain decisions. For a model to be effective, its decisions must be understandable and interpretable, ensuring that both technical and non-technical teams can make informed choices based on its output.
Lastly, there’s the data lag. Timing is everything, and by the time reports are generated, opportunities can pass by. This often happens when businesses wait for perfect data or reports, but freshness matters more than perfection. Statistical models should deliver near real-time insights, allowing companies to adjust marketing campaigns or reallocate resources as needed quickly. Without timely insights, businesses risk missing opportunities or failing to react to emerging challenges.
While these challenges can seem daunting, they’re manageable. By focusing on the right variables, ensuring model transparency, and prioritizing timely insights, businesses can make statistical modeling a powerful tool for decision-making, not a roadblock.
Statistical modeling for the real world
Statistical modeling shifts the focus from asking 'What happened?' to asking 'What’s likely to happen next? ' The businesses that excel with statistical modeling aren’t necessarily the ones with the most data, but those who ask the right questions of the data they have. By shifting the focus from simply understanding past events to anticipating future trends, companies can make smarter, more proactive decisions.
Your data already contains the insights you need to move forward. The real question is whether you’ll continue looking at it through the rearview mirror or start using it to navigate the path ahead. Statistical modeling helps you move beyond past analysis, turning raw data into actionable insights that guide decisions and drive tangible business outcomes.
Statistical modeling empowers businesses to break free from the noise and focus on the signals that matter most. It’s about using data to guide the future, not just reflect on the past.