What Makes For A Successful Analytics Engineer?
Table of Contents
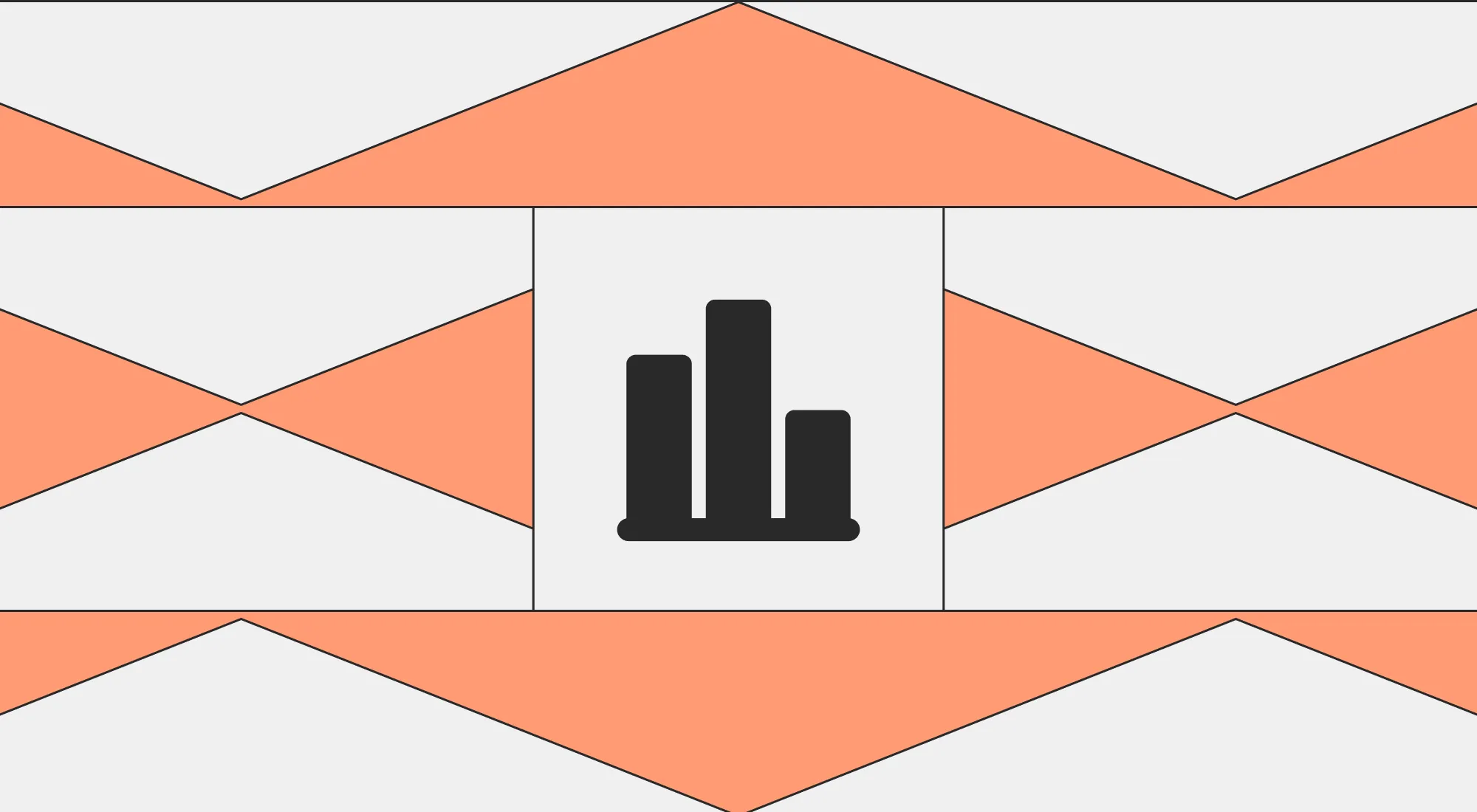
Today’s data and analytics environment is more complex and faster moving than ever before, which means that finding the right person for your team is harder than ever. Even though analytics engineering roles are becoming more common, it is still hard to find the right persona to fit this role. If you’re unfamiliar with what an analytics engineer is, make sure to read this blog post on the Sigma blog. Let’s take a look at some of the attributes and best practices that make for a successful analytics engineer.
What is an analytics engineer?
The rise of low/no-code BI tools like Power BI and Tableau has empowered business users but also introduced challenges in maintaining alignment between Lines of Business (LOBs) and Central IT. Organizations quickly recognized the need for a role that facilitates collaboration between these groups, ensuring both governance and accessibility of data assets. Enter the analytics engineer — a professional uniquely equipped to bridge the divide. Analytics engineers bring a deep understanding of business use cases, fluency in the language of IT, and the technical expertise to align organizational data strategies with real-time analytics needs.
At the heart of the analytics engineer’s role is the ability to navigate the natural gaps between Central IT and LOB users. These gaps arise not from dysfunction but from differing priorities and skill sets. Central IT focuses on the technical aspects of data — ingestion, transformation, and governance — while LOB users prioritize extracting actionable insights to improve business outcomes. Analytics engineers bridge these gaps by acting as translators and collaborators, ensuring that data is both accessible and usable. They enable businesses to strike the delicate balance between IT ownership of data assets and the agility LOBs need to generate meaningful insights.
Technical skills
As we discussed in the opening section of this blog, analytics engineers must be able to communicate effectively with LOB users and Central IT. Let’s dive into some of the technical skills that will make an analytics engineer successful within a data and analytics organization.
Understanding of ETL and data engineering processes
One way that analytics engineers can build credibility with their IT partners is to have a working knowledge of basic data engineering principles. Not only will this be helpful in collaborating with IT, but it will also help your analytics engineer craft solutions that are in line with current IT processes. Additionally, understanding data engineering processes will help ensure that the processes you’re building for the LOB are accurate and that the outcomes are what the LOB actually wants to achieve.
Data modeling
A successful analytics engineer will need to have a very good understanding of data modeling principles and best practices. LOB analysts may be used to seeing spreadsheets with every line of data on one tab, but understanding how to build and scale relational data models will make your IT team happy and benefit the end users in the long run. A good analytics engineer will be able to build a data modeling view that satisfies a use case or two - a GREAT analytics engineer should be thinking about how to create domain-centric data models that satisfy a multitude of use cases, which will make the creation of new analytics from the data assets that much easier.
Low/no-code tools
For rapid prototyping data models, knowledge of low/no-code tools can provide an avenue for quick validation. While these data models will ultimately be pushed back to the CDW in production once they are validated and tested, being able to operate low/no-code tools with expertise will cut down on the time to create the prototypes for the data models. Expertise in these tools will also help accurately identify how to calculate KPIs, validate data, and help explore data and find exceptions to business logic. Additionally, many LOB analysts use these tools when creating their initial self-service analytics as well.
Translate business requirements to technical requirements
While some might see this as more of a soft skill, being able to translate business requirements into technical requirements means having an understanding of business logic, calculations, data processes, and how to create calculations that meet use case requirements — which ultimately is a technical skill. Even if an analytics engineer understands the business logic, they still need to be able to understand how to translate that into whatever syntax you’re using to create the KPI or analytic. The more syntax languages that an analytics engineer is familiar with, the more flexible and effective they will be as the bridge between IT and LOB.
Soft skills
In addition to the technical skills required to be an analytics engineer, it’s also vitally important for an analytics engineer to have a set of “soft skills” for communicating and collaborating with business personas. Let’s dive into some of the soft skills that will make an analytics engineer successful within a data and analytics organization.
Understanding business use cases
To be an effective LOB analyst, you must first understand how your business makes money — or what affects the top and bottom lines of your business income statement. This means that an effective analytics engineer must be able to understand many of the nuances around business sales cycles, cost sources, and how market condition changes impact the business they’re servicing. Without this understanding, an analytics engineer will not have sufficient context around the data to be able to effectively translate requirements into technical requirements, ultimately reducing their effectiveness in serving their LOB stakeholders.
Personable and able to interact with LOB users
The role of an analytics engineer requires a lot of collaboration with many different parties, so in addition to being very strong technically, the role requires a lot of interpersonal skills and the ability to communicate effectively with different personas within an organization. Having strong communication skills and the ability to build strong relationships will only enhance the effectiveness of your analytics engineers. Building strong relationships and being personable to your stakeholders will not only make interactions within the organization more effective, but it will help reduce miscommunications and impact timeliness of deliverables.
Problem solver
This characteristic might seem like the most vague of the ones listed in this section, but it may be the most important for a successful analytics engineer. In the analytics engineer role, receiving very non-technical requirements or requests from your LOB partners is not uncommon. This means that the analytics engineer is often given a very vague starting point, and it’s their job to investigate, comb through data, and work independently to gain an understanding of the LOB asks. This requires a lot of self-driven context gathering, learning, and ultimately problem-solving. There are a lot of pieces to data and analytics puzzles, and many times they are not going to be explicitly stated, so a great analytics engineer must be able to fill in a lot of blanks through their own work.
Attention to detail
Many roles require attention to detail, but it is paramount in the analytics engineer role. When given vague parameters but very specific outcomes, it will be essential to be able to meticulously evaluate how your proposed solution meets business requirements. Because LOB users are not generally experts on each and every table in a CDW, an analytics engineer must be able to comb through this data to ensure that what is being presented in the LOB analytics is accurate and tells the story of the business accurately. This often looks like analytics engineers validating KPIs or data sets to the row and data point level. “Close enough” should not be close enough for a great analytics engineer.
Curiosity
Even though businesses will be certain that one set of reporting requirements they pass on is sufficient for their business needs, an analytics engineer should not only take these requirements at face value; they should be able to have enough context of the business to be able to be proactive in their solution building. This might look like building data views in anticipation of the next set of questions that a business might be asking of their IT partners. This sort of curiosity will make the analytics engineer a technical resource for the business, but it will make them a true partner for the business in achieving their ultimate goals.
How Sigma makes analytics engineers successful
As we’ve discussed, an effective analytics engineer needs to understand both business use cases and technical data processes. Because of these unique requirements, analytics engineers need to leverage tools that allow them to access underlying data from the CDW, visualize data for validation and testing, and drill down to specific data points to investigate discrepancies or validate KPIs. Many tools can do one or some of these, but no tool is built for the niche needs of the analytics engineer more than Sigma.
Sigma’s high-performing direct queries to the CDW allow analytics engineers to work with real-time data without worrying that the data in their model does not reflect the data it has meticulously validated. The easy-to-learn syntax enables analytics engineers to use Sigma to create calculations for business logic and validation purposes quickly. Additionally, Sigma’s drill-through capabilities allow users to investigate individual data points throughout a data product with ease and quickness, which is absolutely necessary for the high-paced day of an analytics engineer.