The Decline of the Business Intelligence Cube (and What’s Replaced It)
Table of Contents
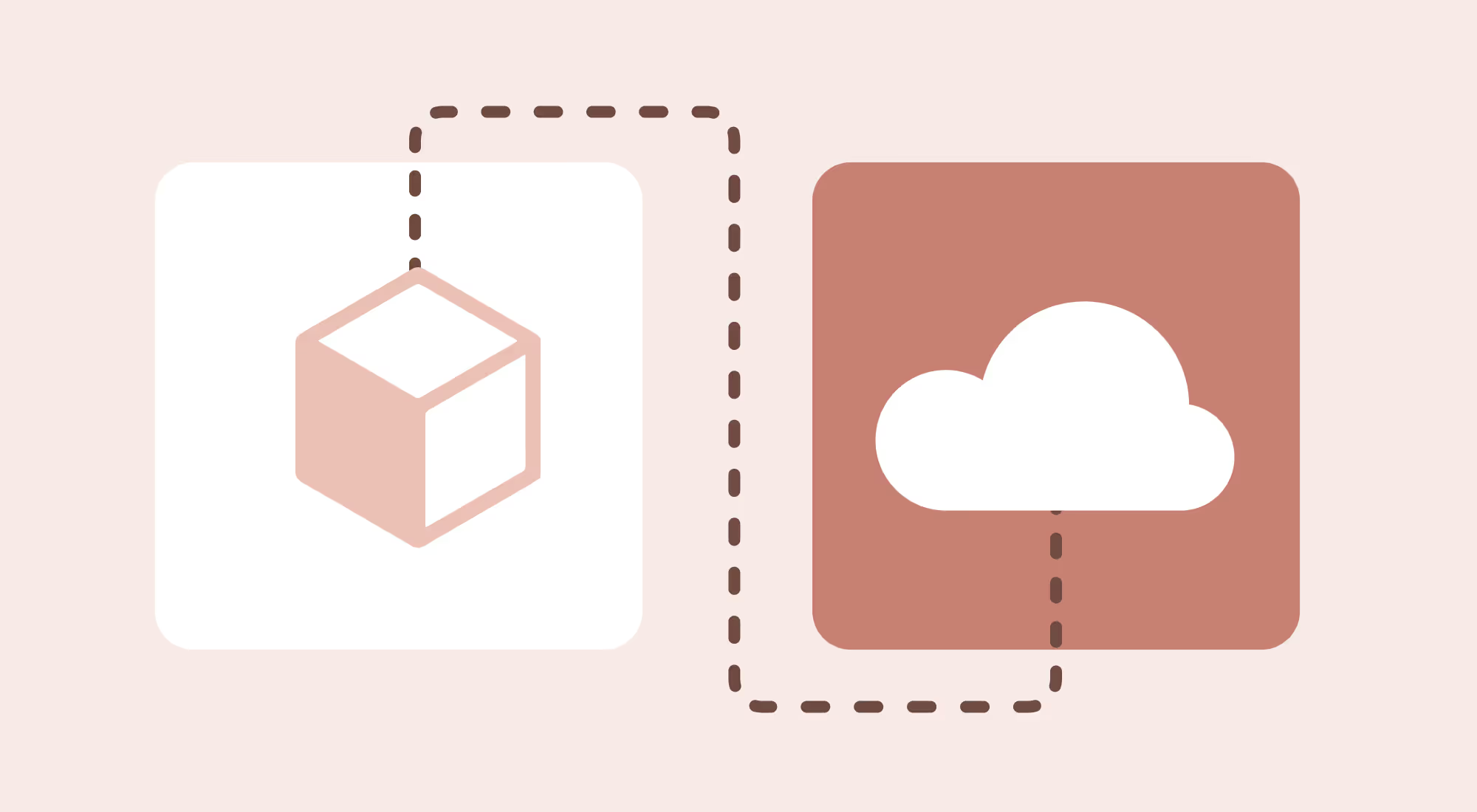
While we’ve come to depend on the power and scalability of the cloud for modern business, it wasn’t that long ago that we didn’t have access to affordable compute power and storage. When servers were on-premises and RAM was expensive to buy, BI teams were limited in the amount of memory they could use to run queries. For this reason, the business intelligence cube, also called a data cube or OLAP (Online Analytical Processing) cube, was developed. Business intelligence cubes enabled teams to take relevant data out of a database and put it into an in-memory data structure for efficient manipulation.
But the relevance of BI cubes has been highly diminished thanks to the cloud and modern data analytics tools. Let’s take a historical look at BI cubes, the developments that made them unnecessary, and the technology that replaced them.
What is an OLAP Cube?
A business intelligence cube or OLAP cube is a data structure optimized for fast and efficient analysis. It enables consolidating or aggregating relevant data into the cube and then drilling down, slicing and dicing, or pivoting data to view it from different angles. Essentially, a cube is a section of data built from tables in a database that contains calculations. Cubes are typically grouped according to business function, containing data relevant to each function. The BI cube was an excellent solution to avoid slow processing times for complex data analysis at a time when compute power was constrained.
Limitations of Data Cubes for Business Intelligence
While BI cubes provided data teams with the ability to run analyses efficiently, they aren’t without significant limitations.
New reports require new cubes or modification
By nature, a business intelligence cube contains only certain dimensions — the dimensions needed when the cube was created for a specific analysis. For example, a report looking at quarterly sales might include location, time, and SKU data. New analyses that include new or different dimensions require that new cubes be created or that the existing business intelligence cube be modified.
ETL pipeline limitations
Because transformation processes require a lot of compute power, and the goal of a business intelligence cube is to provide data ready for analysis, data going into a cube must be transformed prior to loading. Analysts must wait for the BI team to prepare the data and for the transformation process to complete before they can work with the data. Additionally, once data is loaded, it can take significant time to build the cube — between an hour and a full day, depending on the size of the data in the cube. And any time fresh data is needed, the business intelligence cube build process must be run, slowing down decision-making.
Time-consuming modeling
Because a business intelligence data cube must be created or modified each time a new report is needed, data must be organized in the data warehouse in a way that allows it to be quickly accessed and put into a cube. For this reason, data modeling techniques are necessary — but these modeling tasks are time-consuming.
Summarized Information
In an OLAP cube (or business intelligence cube), you can't get to transaction-level detail. Cubes always contain summarized information, so to get to transaction detail, you must leave the cube and go directly against the database. This process is cumbersome, and largely defeats the purpose of using a cube.
Modern Developments Making BI Cubes Unnecessary
Today, most BI teams run OLAP workloads on columnar databases in the cloud rather than creating data cubes as a separate layer. Three developments dramatically reduced the need for business intelligence data cubes: the power and scalability of the cloud data warehouse, the flexibility of modern data pipeline tools, and the way that cloud-native analytics tools connect directly to the CDW.
Power and scalability of the cloud
With the advent of the cloud, compute power and RAM became inexpensive, and resources now easily scale up and down on an as-needed basis. With a modern cloud data warehouse like Google Big Query, Amazon Redshift, Databricks Intelligence Platform, or the Snowflake Data Cloud, organizations can cost-effectively store massive amounts of raw data.
Additionally, with the development of columnar database structure in the cloud data warehouse, BI teams could begin performing OLAP-type workloads at high performance without needing to extract data and build business intelligence data cubes. Practically, this capability allows organizations to perform tasks directly in the data warehouse, without having to create or maintain business intelligence cubes.
Flexibility of SaaS data pipeline tools
Modern SaaS data pipeline solutions such as Fivetran and Matillion offer out-of-the-box connectivity to popular data sources and can also normalize and transform disparate sources of data and move it around without requiring users to write code.
Data exploration and analysis tools connect to the CDW
Today’s BI solutions (like Sigma) connect directly to the cloud data warehouse, taking advantage of the CDW’s power and scalability to access massive amounts of fresh data whenever needed. The best solutions allow decision-makers to directly query live data, down to row-level detail, while maintaining strict data governance. Additionally, users of these tools can traverse data via multiple hierarchies based on the user’s choice, rather than on a rigid pre-modeled exploration experience as business intelligence cubes provide.
Is OLAP Obsolete?
While OLAP cubes (or business intelligence cubes) are now unnecessary, it’s important to note that OLAP workloads are in no way obsolete. OLAP itself enables the flexible multidimensional data analysis that leading organizations use every day. In fact, the Sigma Workbooks is an innovative, direct-manipulation interface enabling visual OLAP at cloud scale.
Modernize Your Business Intelligence
Modernizing your business intelligence involves two key steps. First, take a cloud-native approach to ensure you’re able to take advantage of the power and scalability the cloud offers. Second, take a close look at your organization’s specific business intelligence needs and seek out an analytics solution that meets those needs.
As a cloud-native business intelligence solution, Sigma operates on top of the cloud data warehouse. Because it harnesses the scale, speed, and power of the cloud, it can process data from dozens of sources in seconds. Users can easily crunch through billions of rows of data, and quickly drill into as much detail as needed without the risk of accidentally corrupting or deleting data in the warehouse. With Sigma, teams can analyze billions of rows, create visualizations, join data sources, do rapid what-if analysis, and more via a familiar spreadsheet-like interface.
But sometimes, seeing is believing. Watch how easy it is to build a cubeless pivot table in Sigma in our short demo.
Let’s Sigma together! Schedule a demo today.